=Overview
Humans are capable of building holistic representations for images at various levels, from local objects, to pairwise relations, to global structures. The interpretation of structures involves reasoning over repetition and symmetry of the objects in the image. In this paper, we present the Program Guided Image Manipulator (PG-IM), inducing neuro-symbolic program-like representations to represent and manipulate images. Given an image, PG-IM detects repeated patterns, induces symbolic programs, and manipulates the image using a neural network that is guided by the program. PG-IM learns from a single image, exploiting its internal statistics. Despite trained only on image inpainting, PG-IM is directly capable of extrapolation and regularity editing in a unified framework. Extensive experiments show that PG-IM achieves superior performance on all the tasks.
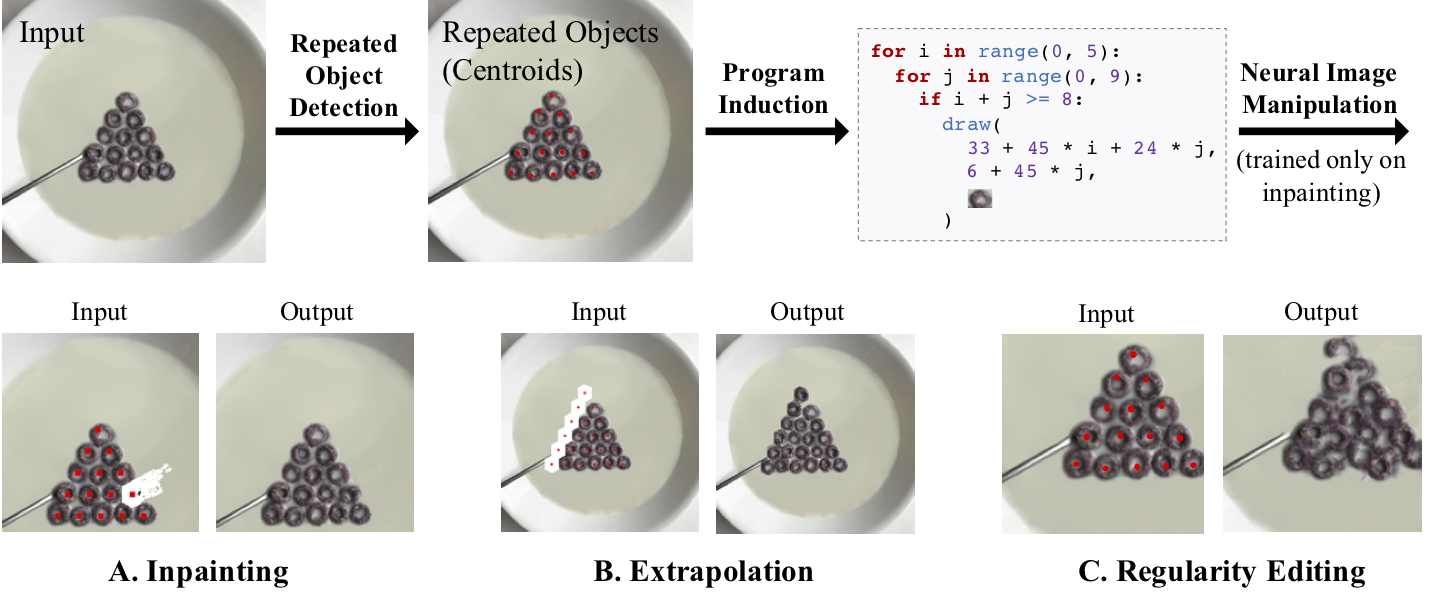
Figure 1: Given an input image, the PG-IM detects repeated entities in the image (pieces of cereal) and then infers a program-like representation for describing the regularity of the image. The regularity representation empowers multiple downstream tasks, such as image inpainting, extrapolation, and regularity editing.